Vilasini Tinnaneri Sreekanth
PHD STUDENT
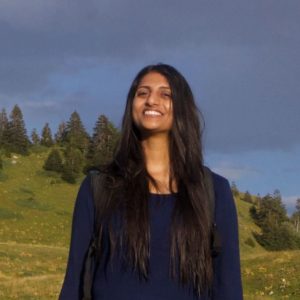
Contact Information | |
E-mail: | vilasini.tinnanerisreekanth [at] cea [dot] fr |
Phone: | |
Office: | 277 |
Affiliation: | IRFU/DAp-AIM |
Supervisors: | Jean-Luc Starck, Sandrine Codis |
Website: | https://vilasinits.github.io/ |
About Me
I am a second year PhD student, working on Likelihood-free parameter estimation using higher-order statistics. I did my Master of Science in Astrophysics from the University of Geneva, Switzerland.
Research Interests
I am interested in understanding the origin and the contents of the universe. My main interest is in large-scale structures, dark energy models, weak lensing, numerical simulations, and machine learning.
The Euclid satellite, to be launched in 2023, will observe the sky in the optical and infrared, and will be able to map large-scale structures and weak lensing distortions out to high redshifts. Weak gravitational lensing mass maps play a crucial role in understanding the evolution of structures in the Universe, distinguishing between dark energy models, and our ability to constrain cosmological models. The traditional method of estimating cosmological parameters using a Gaussian likelihood has several drawbacks. To overcome this, a new approach has recently emerged, called likelihood-free cosmological parameter inference, which is based on forward modeling, bringing with it a possibility to use high-order statistical information, and it is relatively straightforward to include all systematic effects. With this, we do not need covariance matrices anymore and avoid the storage of huge simulated data sets. But this method also has drawbacks, the first one is the need for huge GPU resources to process surveys such as Euclid and the second is that the solution relies on the accuracy of simulations. In another recent breakthrough (Codis,2021), we now have theoretical tools to predict, for a given set of cosmological parameters, the multiscale density probability function (PDF) of convergence maps such as the one that will be observed with Euclid.
The goal of my PhD is to develop a hybrid approach, consisting of a likelihood-free cosmological parameter inference based on the high-order statics theoretical prediction rather than n-body simulations. This approach will have the advantage of not needing to store huge data sets to compute a covariance matrix, and not requiring huge CPU/GPU resources as the forward modeling method, making this method highly competitive to constraint the cosmological model using high order statistics in future surveys.
I am a member of EUCLID collaborations.
I am also one half of the CosmoStat Journal Club organizing committee; if you would like to give a seminar at the lab, please feel free to contact Lisa , Ezequiel or me!
Conferences, Schools and Talks
- Euclid-France Theory and Likelihood Workshop, Nov 28th 2022, IAP Paris
- Euclid France Meeting 2022, Nov 30th-Dec 2nd 2022, IAP Paris
- XV Tonale Cosmology Winter School, Dec 4th-10th 2022, Passo del Tonale, Italy
- Future Cosmology, Ecole themathique du CNRS, April 23-29 2023, Cargese, France (Poster)
- ADA X Summer School, Sept 18-22 2023, Hersonissos, Crete, Greece
- Colloque national Action Dark Energy, Nov 06-07 2023, Annecy, France (Talk)
- TOSCA reunion meeting, Nov 16-17 2023, Nice, France (Talk)
- Euclid-France Symposium 12 - 24-26 2024, Lyon, France (Talk)
- Cosmology and Statistics Days, 01-02 February, CosmoStat, CEA Paris-Saclay, France (Talk)
- Euclid-SWG WL meeting 19-21 February, 2024, Innsbruck, Austria (Talk)
Education
- Master of Science in Astrophysics, University of Geneva, Switzerland, 2021
- Integrated Master of Science in Physics, S V National Institute of Technology, Surat, India, 2019
Master Thesis
For my master's thesis at the University of Geneva, I worked under the guidance of Prof. Martin Kunz and Dr. David Daverio on N-body simulations of cosmic strings to study their impact on structure formation. In this project, I have developed a field-theoretical simulation of global $O(N)$ defects which is based on the framework of LATField2, and then incorporated this within a relativistic N-body simulation code Gevolution. After performing a few tests to cross-validate and interpret the results, the defect stress-energy-tensor was added to the matter stress-energy-tensor in the metric solver for the relativistic N-body code and the effect on scalar modes were analyzed.