Tobias Liaudat
RESEARCH ENGINEER
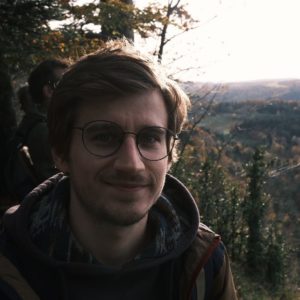
Contact Information | |
E-mail: | tobias.liaudat [at] cea [dot] fr |
Affiliation: | IRFU |
Website: | https://tobias-liaudat.github.io |
You can find more up-to-date info on my website: tobias-liaudat.github.io
About me
I am currently a PhD student working on the crossroads of Inverse Problems, Machine Learning and Cosmology. I enjoy including the underlying physics of the task into the Inverse Problem with the help of Machine Learning in order to build novel and better methodology that can tackle the challenges raised in Cosmology by forthcoming missions.
Research Interests
The main motivation of my PhD is to develop a data-driven model for Euclid’s space telescope instrumental response, or Point Spread Function (PSF), that will account for spatial, spectral and temporal variations. The model should be able to estimate Euclid’s PSF at any position of the satellite’s Field of View (FoV) in order to be able to correct its effect on the observations. Taking into account the PSF is a mandatory if one wants to do weak gravitational lensing that is not unacceptably biased. The PSF constitutes one of the main sources of systematics in weak lensing studies.
The Euclid space telescope, to be launched in 2023 from the European Space Agency (ESA), will be able to observe the sky in the optical and infrared, and will be able to map large scale structures and weak lensing distortions out to high redshifts. Weak gravitational lensing is a fundamental tool of cosmology to constrain models. For the mission to be successful the measurements have to be very precise and, therefore, it is vital to have a reliable PSF model.
I am also working on a data-driven PSF model for ground-based telescopes. I developed a new modelling method that can make a model simultaneously on the full focal plane, incorporating the geometry of the instrument. I validated the approach with real data from the CFIS survey at the Canada France Hawaii Telescope (CFHT), and integrated the model into a high performance shape measurement pipeline.
My main interests are Inverse problems, Machine Learning, Image and signal processing, Point Spread Function modelling, weak lensing, and convex and non-convex optimisation.
Master thesis
I did my Master thesis in the CosmoStat laboratory under the supervision of Jérôme Bobin and Christophe Kervazo. We developed a new method coined Distributed GMCA (DGMCA) for tackling large scale sparse Blind Source Separation (BSS) problems where we are not able to scan all the observations simultaneously. DGMCA is able to parallelise the workload by the use of a clever manifold-based matrix aggregation procedure. It is able to accelerate the widely-used BSS algorithm GMCA (Generalised Morphological Component Analysis) while maintaining the separation performance. On top of that, it can even outperform GMCA under some conditions.
Peer-reviewed scientific journals
- Shapepipe: a new shape measurement pipeline and weak-lensing application to UNIONS/CFIS data.
A. Guinot, et al. (including T. Liaudat)
Submitted to A&A. (2021) - Multi-CCD Point Spread Function Modelling,
T. Liaudat, J. Bonnin, J.-L. Starck, M.A. Schmitz, A. Guinot, M. Kilbinger, S.D.J. Gwyn.
A&A, 646:A27. 2021. (DOI, code) - Faster and better sparse blind source separation through mini-batch optimization,
C. Kervazo, T. Liaudat, J. Bobin.
Digital Signal Processing, Elsevier 2020. (DSP, HAL)
Peer-reviewed conference proceedings
- Rethinking the modeling of the instrumental response of telescopes with a differentiable optical model.
T. Liaudat, J.-L. Starck, M. Kilbinger.
NeurIPS 2021, Machine Learning and the Physical sciences workshop. (conference, ArXiv, poster) - Semi-parametric wavefront modelling for the point spread function.
T. Liaudat, J.-L. Starck, M. Kilbinger.
52èmes Journées de Statistiques de la Société Française de Statistique (SFdS). - Distributed sparse BSS for large-scale datasets,
T. Liaudat, J. Bobin, C. Kervazo.
SPARS, Toulouse, France, July 1-4, 2019 (Article, Poster, code)
Talks
- 2021 NeurIPS Machine Learning and the Physical Sciences Workshop (Poster). Online, December 2021.
- Journée de lancement de l'axe Astrophysique de la Graduate School Physique. Semi-parametric wavefront modelling for the point spread function. Saclay, France, November 2021.
- 52èmes Journées de Statistiques de la Société Française de Statistique. Semi-parametric wavefront modelling for the point spread function. Online, June 2021.
- Peyresq summer school on signal and image processing. Online, June 2021.
- 2021 UNIONS CFIS/Pan-STARRS/WISHES Collaboration Meeting. Multi-CCD PSF modelling method. Online, March 2021.
- SPARS 2019 conference (Poster). Toulouse, France. July 2019.
- CosmoStat's seminar. Optimal transport for signed measures. Saclay, France, February 2019.
Teaching
- 2020-2021: Teaching assistant within the applied mathematics department DepMap from École Polytechnique.
- Statistics [MAP433] for M2 students with Prof. Eric Moulines.
- Optimisation and control [MAP435] for M2 students with Prof. Grégoire Allaire.
- Mathematical modelling [MAA107] for L1 students with Prof. Vincent Bansaye and Prof. Thibaut Mastrolia.
- Applied mathematics python projects [MAP361P] for M1 students with Prof. Arvind Singh. - 2021-2022: Teaching assistant within the applied mathematics department DepMap from École Polytechnique.
- Optimisation and control [MAP435] for M2 students with Prof. Grégoire Allaire.
- Applied mathematics python projects [MAP361P] for M1 students with Prof. Arvind Singh.
Education
- Masters II SISEA in Research, specialised in Image Processing, IMT Atlantique, Brest, France, September 2018.
- Engineering degree, specialised in Image Processing, IMT Atlantique, Brest, France, September 2018.
- Electronic engineering degree, Faculty of Engineering, University of Buenos Aires, Argentina, September 2019.
Supervision
- Aziz Ayed - Master student, 6 month internship at CosmoStat (2020)
Joint supervision with Jean-Luc Starck.
Working on deep denoisers for the MCCD PSF model. - Jérôme Bonnin - Master student, 5 month internship at CosmoStat (2019)
Joint supervision with Jean-Luc Starck.
Working on RCA for CFIS and on the MCCD PSF model.
Collaborations
I am part of the Euclid Consortium and the UNIONS/CFIS collaboration.
Reviews
I reviewed papers for the following journals:
- Astronomical Journal from AAS (1 research article).
Other links
Miscellaneous
I am one of the organisers of CosmoStat's CosmosClub that is our Journal club/Group seminar. If you would like to give a talk and meet the group do not hesitate to contact me.
- Click here to learn recursion.